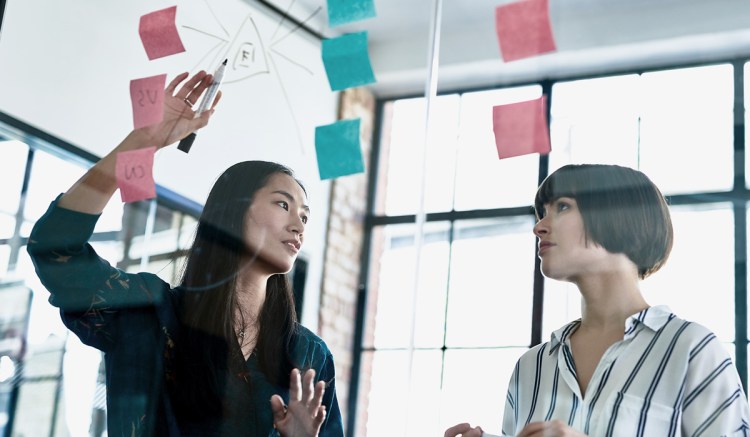
What do explainability and compliance have in common? It’s in the ‘AI’
As global regulatory bodies move to explore the implications of ChatGPT and other large learning models, we speak to Global Relay’s in-house experts about the importance of explainability.
Written by a human
At the beginning of April 2023, Elon Musk and other technology luminaries called for a halt to the training of certain AI while governance systems are “rapidly accelerated”. In the same week, the UK government published a Policy Paper looking to put the UK “on course to be the best place in the world to build, test, and use AI technology”.
Since that blog was written, and in this week’s edition of ‘the rollercoaster that is generative AI’, a number of government bodies have announced that they are probing ChatGPT and its maker, Open AI, over suspected breaches of the European Union’s General Data Protection Regulation (GDPR).
Firstly, Italy’s data protection authority – Garante Per La Protezione Dei Dati Personali (GPDP) – enforced a ban on ChatGPT on the grounds that “personal data is collected unlawfully”. The GPDP enforced an immediate-yet-temporary ban on the processing of Italian users’ data by OpenAI while an inquiry was initiated. In particular, it expressed concern that “there appears to be no legal basis underpinning the massive collection and processing of personal data in order to ‘train’ the algorithms on which the platform relies”.
On April 11, 2023, France’s privacy watchdog, the CNIL, said that it was investigating “several complaints” about ChatGPT. Two days later, it was the turn of the Spanish Data Protection Authority (AEPD) to address the data concerns posed by OpenAI, similarly announcing a preliminary investigation into its data processes. Unlike the Italian data authority, it does not appear that Spain has blocked the service in its country.
On the same day that Spanish authorities announced concerns about ChatGPT, the heavy hitters waded in, with the European Data Protection Board announcing that it has launched a dedicated task force to “foster cooperation and to exchange information on possible enforcement actions conducted by data protection authorities”.
Explain or expend
For those to whom data lies close to their heart, these developments likely come as no surprise. It has been widely circulated that OpenAI’s own FAQs acknowledge that the data submitted by users into ChatGPT can be reviewed by OpenAI staff and contractors. The capture, storage, retention, and ownership of data plugged into ChatGPT has long been a concern, and data protection agencies now appear to be catching up.
Recent movement from regulatory bodies is symbolic of a growing industry reaction to artificial intelligence. Like Icarus, the adoption of AI has been treated with impatience – firms have acted fast to innovate, adopt, and implement AI-derived processes – but now they’re flying dangerously close to the sun.
Artificial Intelligence is, of course, hugely beneficial to financial services. But firms should take a considered approach to how they use it. The key to success? Explainability.
Global Relay’s Director of Regulatory Intelligence, Rob Mason, has experienced first-hand the evolution of artificial intelligence for use within compliance teams.
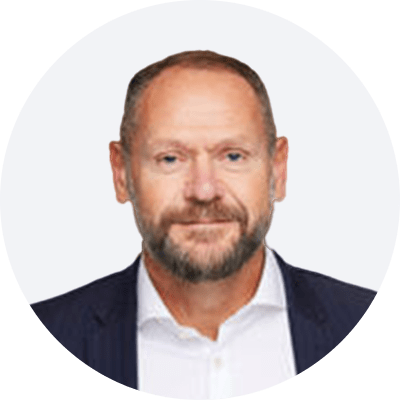
One of the key challenges faced by the compliance surveillance officer when using AI as part of their monitoring solution is explainability.
Due to the heavy burden of regulation in this area, in conjunction with most organizations’ strong governance and oversight, every element of every control needs to be carefully documented and explained. Typically, a change to alerting would need to pass through a governance process which might include internal senior stakeholders, model validation teams, internal audit teams, external audit teams and, of course, the regulators themselves.
The benefits that AI brings have great potential and some vendors have framed the surveillance challenge by suggesting that unless you are at the forefront of utilizing and delivering cutting-edge AI to identify new risks, then you are nowhere. Despite massive resource effort and while some progress has been made, the truth has so far been noted that those vendors have materially overpromised and significantly underdelivered in effectively identifying previously unseen risks.
One of the key hurdles to overcome is explaining how artificial intelligence and machine learning actually works to evolve the controls which are running. For example, an alert will identify something as suspicious on one day, but due to changing circumstances on another day it does not.
While there may be advantages to this approach, there needs to be a clear, easily explainable rationale for why this has happened and also that the change has been validated.It may be fair to say that this is slowing the progress that can be made in this space, but such is the scrutiny placed around regulatory alerting, that if a single alert fails to fire (when it should have), the consequences can be material.
Typically, some firms may use artificial intelligence to identify communication types which they may decide to exclude from the scope of supervision e.g. whitelist, broad circulation emails (in excess of 12 addressees), circulars, blanket coverage communications, or others which represent zero risk of abuse – this can hugely reduce the number of communications being included in the review process and so ultimately reduce the number of false positives which this may create. This can be reasonably documented and explained.Where an algorithm is used to determine a scoring methodology on levels of suspicion, it is technically challenging to identify ‘same case scenarios’ i.e. where similar rules can be applied to another communication so the outcome is successfully repeated. Feeling confident (based on a statistically significant sample of test data) has proven difficult to deliver and challenges of inconsistent monitoring have not been overcome.
Ultimately, risk-based compliance is founded around a principle of building a robust position with evidence that can be defended if rigorously challenged.
AI needs to be deployed, tested and used carefully to meet this requirement.”
Approach with caution
The power of artificial intelligence, especially that of generative AI and large language models, should not be understated. Neither should the risks of deploying AI without careful consideration. Ask first, can you clearly establish and explain how a process has been conducted using AI?
Global Relay’s Chief Data Scientist, Matt Barrow, added:
If a model does not manage all related data in a secure way and does not produce predictions in an explainable and interpretable way then it is not fit-for-purpose.
Model opacity is a real issue in compliance and comes with a trade-off. The most transparent and therefore explainable of models would be a simple lexicon. The most opaque would be a large language model. The trade off is accuracy, with the latter outperforming the former but being highly unexplainable to stakeholders.
The optimal level of acceptable model opacity needs to be discovered by the relevant compliance professionals. Starting with simple, explainable models and gradually adding more sophisticated (and opaque) models until a certain level should be part of an institutions model governance and risk management programming.“
At Global Relay, we use AI to underpin our compliance offering, from intelligent archiving to surveillance and supervision. We are considered in our approach, ensuring that explainability is front-and-center. We build our own technology from the ground up, so we understand where it comes from, how it works, and why. So you can harness cutting-edge technology, but mitigate the risk of the unknown.